Introduction
Retrieval-Augmented Generation (RAG) combines the power of AI with advanced search methods to find important information from your internal documents and external data sources. It uses a pre-trained Large Language Model (LLM) to turn this information into clear, personalized responses. This technology lets businesses modify Large Language Models (LLMs) for different needs, creating a range of specialized AI assistants. For example, a GPT model designed for sales can create effective sales strategies and customer engagement plans. A GPT for research and development could help with innovation and technical analysis. Also, a GPT focused on customer service can improve how queries are resolved and enhance interactions with clients.
Watch our demo to see how AMAX uses a RAG trained on our products for efficient internal document searches.
Understanding Retrieval-Augmented Generation (RAG)
What is RAG?
Retrieval-Augmented Generation stands at the forefront of AI technology, integrating generative AI with advanced embedding techniques. This combination enables AI to access and utilize external datasets, refining the information with a pre-trained Large Language Model (LLM) into accurate, custom responses. The capability of RAG to process and analyze extensive datasets makes it an essential tool for businesses seeking to enhance their AI capabilities.
Customized AI Solutions for Various Departments
RAG's adaptability allows for its application across various organizational departments. A RAG-tailored GPT model for marketing, for example, could generate innovative copy and devise content strategies. Conversely, a finance-focused GPT might specialize in complex economic analysis and data management. In human resources, RAG could streamline recruitment and bolster employee engagement strategies, showcasing its broad applicability.
Key Benefits of RAG
RAG presents numerous advantages for enterprises:
- Up-to-date Insights: Enables real-time information retrieval, offering current and contextually relevant responses.
- In-House Data Protection: Enhanced security within your network ensures data confidentiality.
- Custom Configuration: Tailored to meet specific business needs, offering bespoke solutions.
- Reliable Performance: Guarantees consistent, exclusive performance for your enterprise.
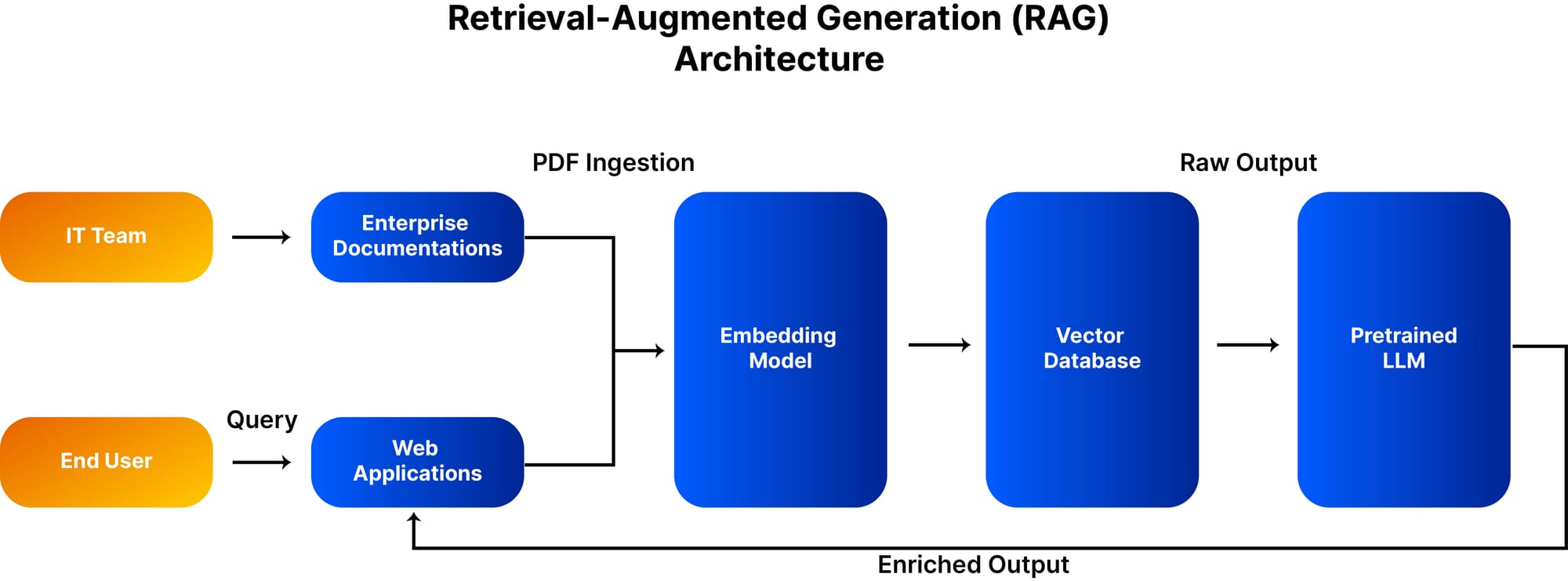
RAG Architecture
The architecture of RAG comprises several components like the embedding model, vector database, and pretrained LLMs. This structure is designed to efficiently process enterprise documentation, ensuring enriched and tailored output for end users.
Enhancing Your LLM with RAG
Incorporating RAG into LLM deployment enhances functionality, allowing secure integration with internal business data. An on-premises RAG solution ensures that queries and data remain within the local infrastructure, offering security against cloud vulnerabilities. RAG-enhanced LLMs offer real-time data sourcing, yielding current, contextually relevant responses and minimizing sensitive data exposure risks.
AMAX's On-Prem Engineered Solutions for AI
AMAX offers on-prem solutions augmented with RAG technology, providing businesses with secure, efficient, and customized AI experiences. These solutions cater to specific enterprise needs, ensuring data security and performance reliability.
Retrieval-Augmented Generation is reshaping enterprise AI, providing tools that can be tailored to various business functions. Its capabilities in providing up-to-date insights, data protection, and reliable performance make it an indispensable asset for modern businesses. As enterprises navigate the complexities of the digital age, RAG emerges as a beacon of innovation, driving efficiency and informed decision-making.